About Me
Hi, I'm Jui.
I am looking for MLE roles (or now AI roles) or SDE in ML/AI starting May 2023.
I have a strong research background in AI with a few publications with great amount of
citations for a New Grad (52 on Google Scholar) and have collaborated for papers with
research labs and researchers from Bloomberg and DeepMind. Also, have had internships
at Linkedin and Deutschebank.
I'm Seeking FTE roles starting May 2023.
Publications Resume Google Scholar
Publications
What all do audio transformer models hear? Probing Acoustic Representations for Language Delivery and its Structure
- Preprint on arxiv - 22 citations
- 2021
- Paper
In recent times, BERT based transformer models have become an inseparable part of the 'tech stack' of text processing models. Similar progress is being observed in the speech domain with a multitude of models observing state-of-the-art results by using audio transformer models to encode speech. This begs the question of what are these audio transformer models learning. Moreover, although the standard methodology is to choose the last layer embedding for any downstream task, but is it the optimal choice? We try to answer these questions for the two recent audio transformer models, Mockingjay and wave2vec2.0. We compare them on a comprehensive set of language delivery and structure features including audio, fluency and pronunciation features. Additionally, we probe the audio models' understanding of textual surface, syntax, and semantic features and compare them to BERT. We do this over exhaustive settings for native, non-native, synthetic, read and spontaneous speech datasets.
MSPEC-NET : MULTI-DOMAIN SPEECH CONVERSION NETWORK
- 45th International Conference on Acoustics, Speech, and Signal Processing, ICASSP 2020
- IEEE | May 4-8,2020 | 4 citations
- Paper | Demo | Code
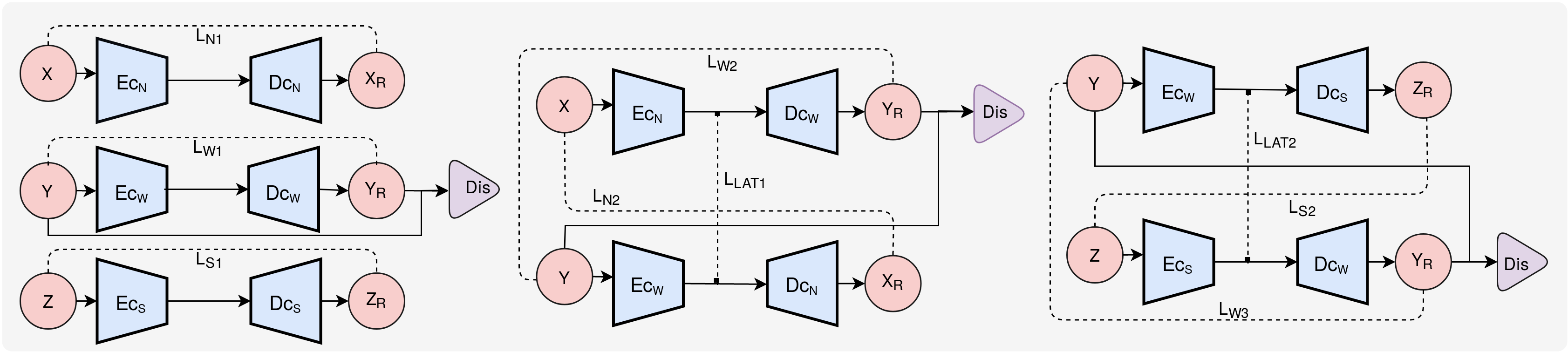
In this paper, we present a multi-domain speech conversion technique by proposing a Multi-domain Speech Conversion Network (MSpeC-Net) architecture for solving the less-explored area of Non-Audible Murmur-to-SPeeCH (NAM2-SPCH) conversion. The murmur produced by the speaker and captured by the NAM microphone undergoes speech quality degradation. Hence, NAM2SPCH conversion becomes a necessary and challenging task for improving the intelligibility of NAM signal. MSpeC-Net contains three domain-specific autoencoders. The multiple encoder-decoders are aligned using latent consistency loss in such a way that the desired conversion is achieved by using the source encoder and target decoder only. We have performed zero-pair NAM2SPCH conversion using the interaction between source encoder and the target decoder. We evaluated our proposed method using both objective and subjective evaluations. With a Mean Opinion Score of 3.26 and 3.12 on an average in a direct NAM2SPCH, and an indirect NAM2SPCH (i.e., NAM-to-whisper-to-speech) conversion, respectively. MSpeC-Net achieves the perceptually significant improvement for NAM2SPCH conversion system.
CinC-GAN for Effective F0 predictionfor Whisper-to-Normal Speech Conversion
- 28th European Signal Processing Conference (EUSIPCO), IEEE
- IEEE | Jan 18-22,2021 | 2 citations
- Paper
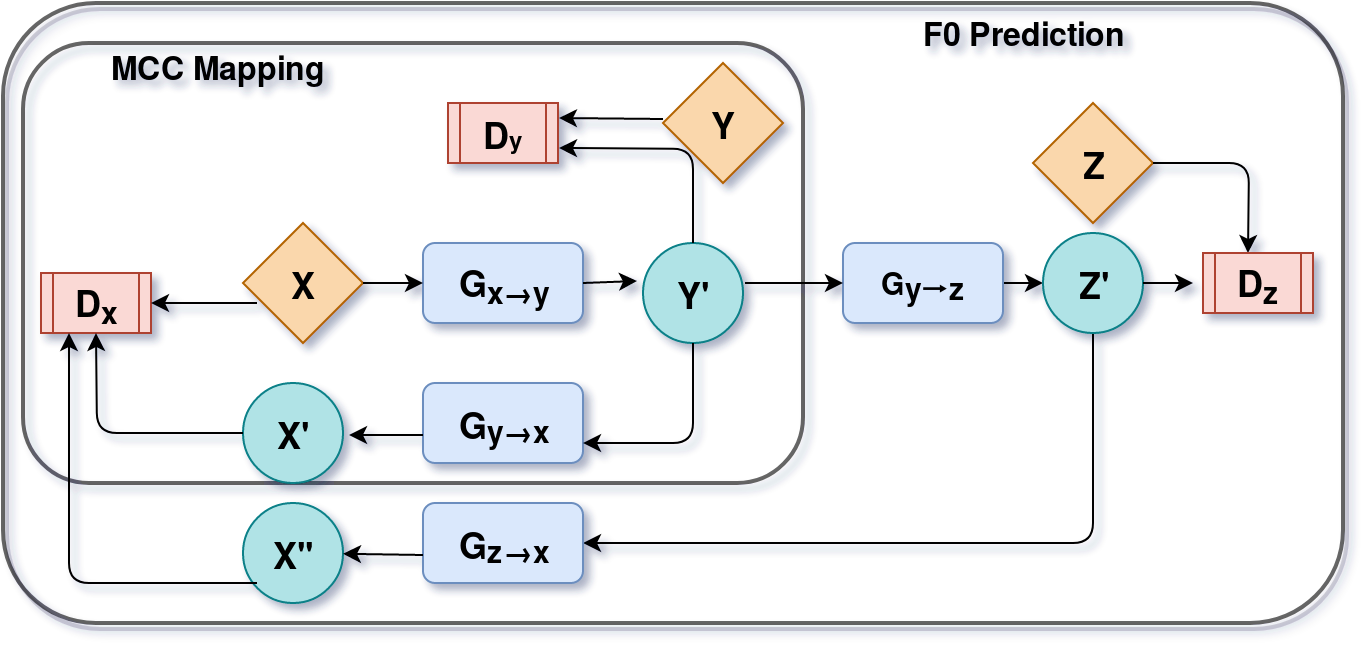
Recently, Generative Adversarial Networks (GAN)-based methods have shown remarkable performance for the Voice Conversion and WHiSPer-to-normal SPeeCH (WHSP2SPCH) conversion. One of the key challenges in WHSP2SPCH conversion is the prediction of fundamental frequency (F0). Recently, authors have proposed state-of-the-art method Cycle-Consistent Generative Adversarial Networks (CycleGAN) for WHSP2SPCH conversion. The CycleGAN-based method uses two different models, one for Mel Cepstral Coefficients (MCC) mapping, and another for F0 prediction, where F0 is highly dependent on the pre-trained model of MCC mapping. This leads to additional non-linear noise in predicted $F_0$. To suppress this noise, we propose Cycle-in-Cycle GAN (i.e., CinC-GAN). It is specially designed to increase the effectiveness in F0 prediction without losing the accuracy of MCC mapping. We evaluated the proposed method on a non-parallel setting and analyzed on speaker-specific, and gender-specific tasks. The objective and subjective tests show that CinC-GAN significantly outperforms the CycleGAN. In addition, we analyze the CycleGAN and CinC-GAN for unseen speakers and the results show the clear superiority of CinC-GAN.
Effectiveness of Transfer Learning on Singing Voice Conversion in the Presence of Background Music
- International Conference on Signal Processing and Communication (SPCOM) 2020
- IEEE | Jul 20-23,2020 | 2 citations
- Paper
Singing voice conversion (SVC) is a task of converting the perception of source speaker’s identity to target speaker without changing lyrics and rhythm. Recent approaches in traditional voice conversion involves the use of the generative models, such as Autoencoders (AE), Variational Autoencoders (VAE), and Generative Adversarial Networks (GANs). However, in case of SVC, GANs are not explored much. The only system that has been proposed in the literature u ses traditional GAN on the parallel data. The parallel data collection for real scenarios (with the same background music) is not feasible at all. Moreover, SVC in the presence of background music is one of the most challenging tasks as it involves the source separation of vocals from the inputs, which will have some amount of noise. Therefore, in this paper, we propose transfer learning, and fine-tuning-based Cycle consistent GAN (CycleGAN) model for non-parallel SVC, where music source separation is done using Deep Atrractor Network (DANet). We designed seven different possible scenarios to identify the best possible combination of transfer learning and fine-tuning. Here, we use more challenging database, MUSDB18, as our primary dataset. And, we use NUS-48E database to pretrain CycleGAN. We perform extensive analysis via objective and subjective measures and report that with a 4.14 MOS score out of 5 for naturalness, the CycleGAN model pretrained on NUS-48E corpus performs the best compared to the other systems we have described in the paper.

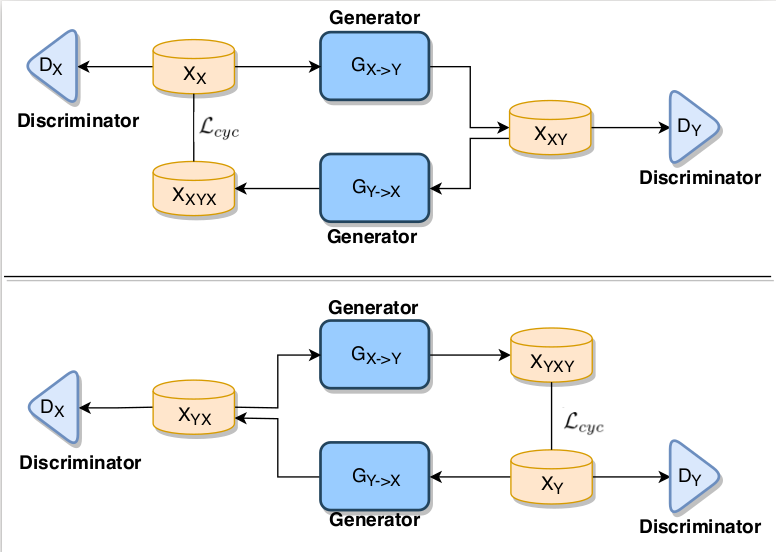
Real-Time Neural-Net Driven Optimized Inverse Kinematics for a Robotic Manipulator Arm
- International Conference on Advanced Machine Learning Technologies and Applications (AMLTA) 2020
- Springer | Feb 13-15,2020 | 1 citation
- Paper
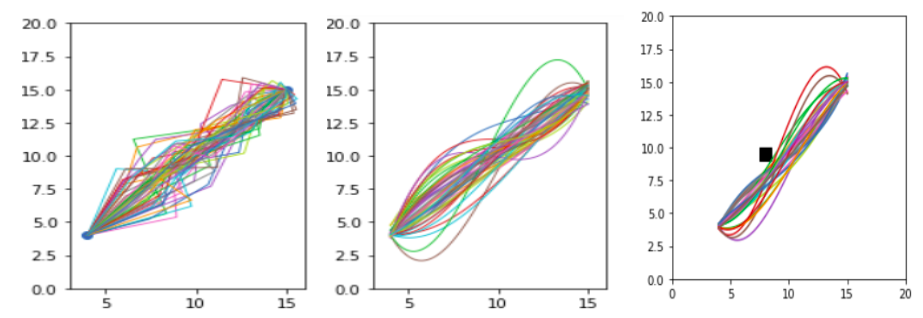
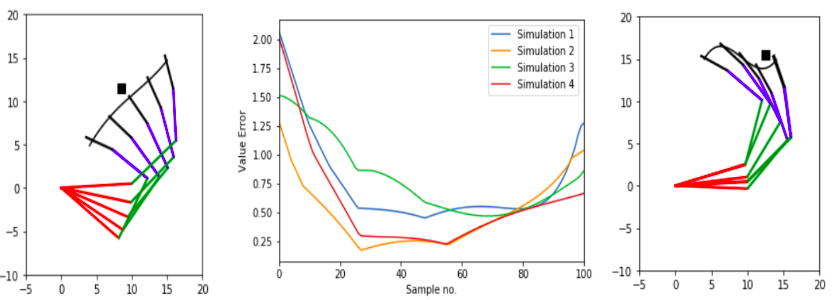
This paper proposes a method that optimizes the inverse kinematics needed for the trajectory generation of a 4-DOF (degrees of freedom) robotic manipulator arm to give results in real time. Due to the many-to-one mapping of the angle vector which describes the position of the manipulator joints and to the coordinates of the end-effector, traditional methods fail to address the redundancy that exists in an efficient way. The proposed method is singular, and in that it (1) Generates the most optimal angle vector in terms of maximum manipulability, a factor which determines the ease with which the arm moves, for a given end-vector. (2) Proposes a novel approach to inculcate the complexity of dealing with real coordinate system by proposing a machine learning technique that uses neural networks to predict angle vector for practically any end-effector position although it learns on only a few sampled space. (3) Works in real time since the entire optimization of the manipulability measure are done offline before training the neural network using a relevant technique which makes the proposed method suitable for practical uses. (4) It also determines the shortest, smooth path along which the manipulator can move along avoiding any obstacles. To the best of the authors’ knowledge, this is the first neural-net-based optimized inverse kinematics method applied for a robotic manipulator arm, and its optimal and simple structure also makes it possible to run it on NVIDIA Jetson Nano Module.
Connect @
Email:
shahjui2000@gmail.com
University Email:
jui_shah@daiict.ac.in